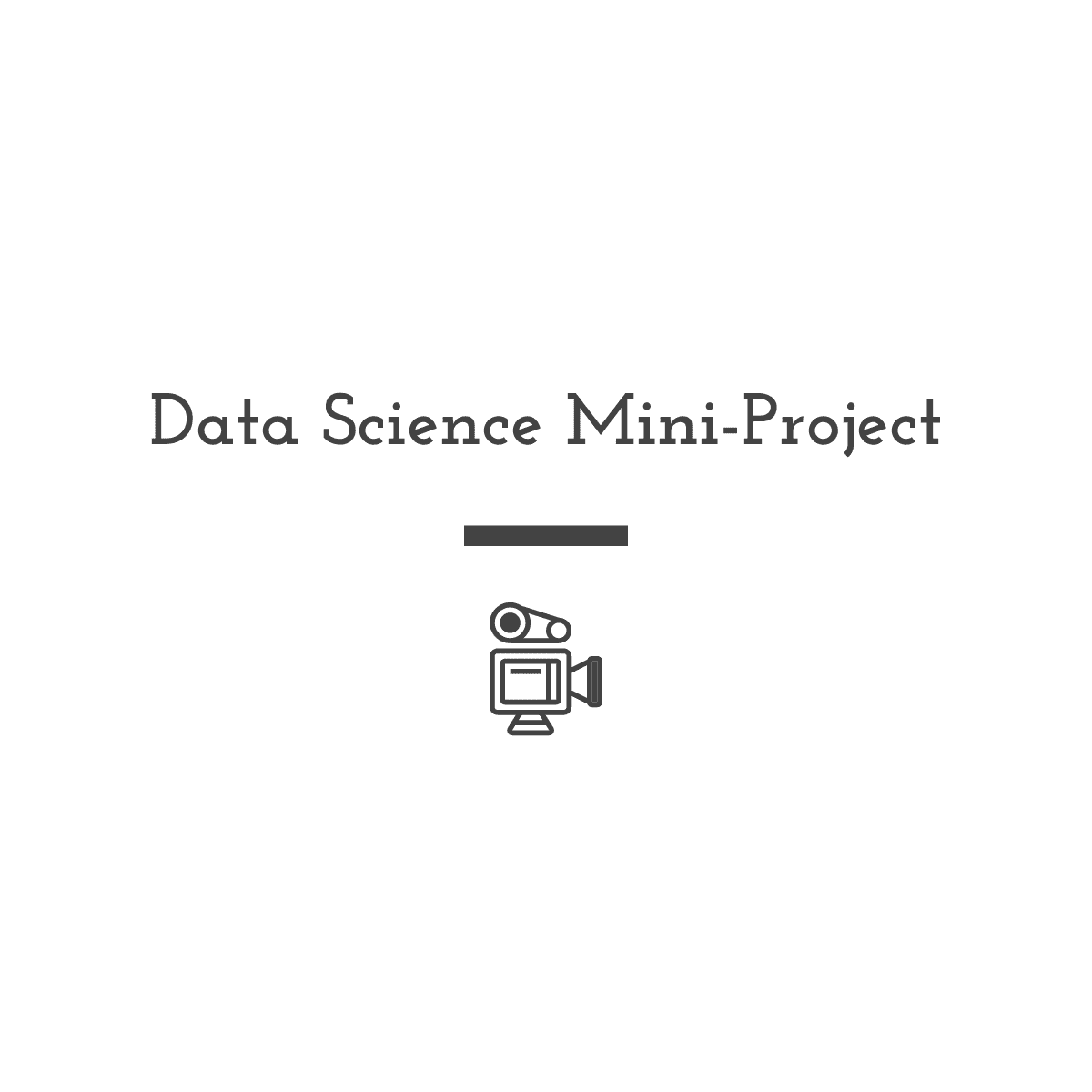
Project Overview:
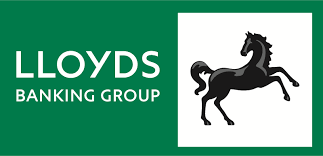
The project deals with two synthetic datasets provided by Lloyds Banking Group (LBG) containing bank transactional data that is very similar to real-world data. Performing an exploratory data analysis on these datasets can help answer several compelling questions that could reveal useful insights for decision-makers, such as: understanding customer behaviour, average customer spending, identifying peak spending months, which shops have the highest spending and whether customers that spend more tend to transfer money to other people, who also spend more.
Our main contribution is analysing two synthetic datasets, consisting of 13234863 million transactional records and 174601 transactional records, respectively.
This dataset was combined with insights from the analysis, which included a dashboard, representing a detailed customer transaction for money payed into the customer's account and money paid out of the account, along with details of the counter-party to the transaction.
We also applied unsupervised and supervised learning techniques, including data clustering and building a decision tree classification model, to classify customers into four different groups with similar characteristics, based on the customer's spending density and transfer frequency.
We found that the customers with the highest spending frequency in terms of transactions had been spending their money in bars or pubs. Moreover, we found that customer spending increased at the start of the year (January) and at the end of the year (December).
We concluded that banks should classify customers according to their profits and loyalty to the bank when recommending suitable financial products to them with corresponding service quality. To create this recommendation engine, we have built a classification model that divides all customers into four groups: the ordinary customer, loyal customer, potential valuable customer and priority customer.
Sample Report
Review A Sample of The Report in PDF Format